Data-driven insights are a real supercharger for a wealth advisory business. The main ingredient, the data, is already in-house and waiting to be uncovered. However, most banks and wealth managers face the complexity and incalculable difficulties of the task early on in their data science journey. With this blog, we help you align your high-flying expectations with the sometimes-harsh reality of data science implementation.
Break down your goals into tangible data science use cases
The importance of data analytics and the potential benefits for financial institutions are undisputed facts. However, it is crucial to set clear targets at the beginning of a data science undertaking and to capture them in practical use cases. As a report by BCG [1] revealed, advanced analytics have the potential to substantially increase assets under management, client acquisition and retention. However, business growth is not the only benefit data science can deliver to an organization. Personalization of services, efficiency gains and risk reduction should not be neglected.
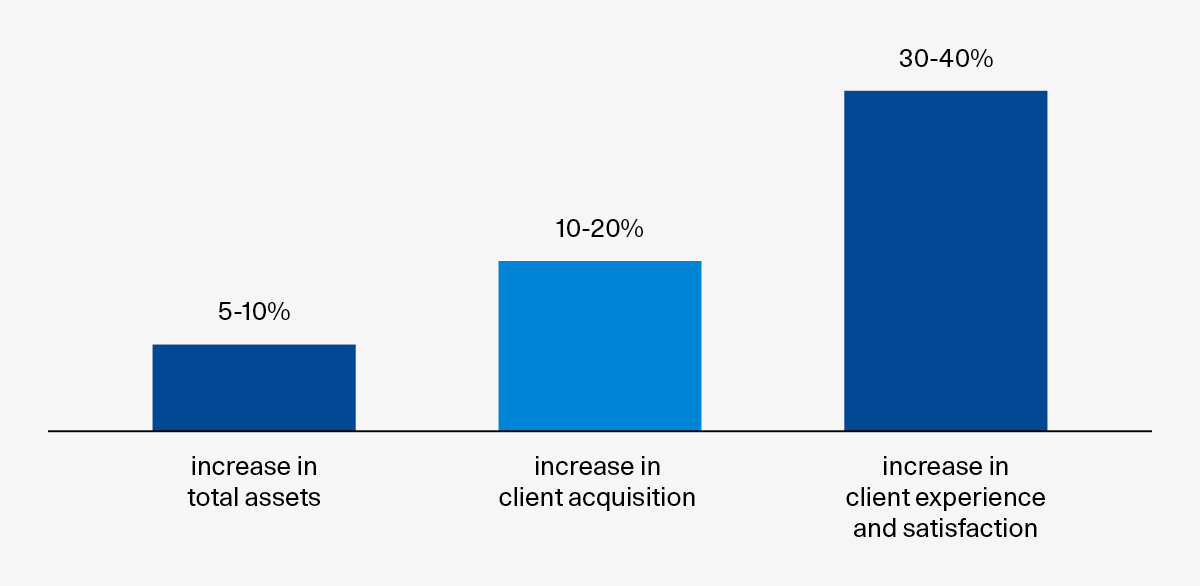
In another blog post, we highlighted the potential of relationship networks and translated this data science application into use cases to help relationship managers identify new prospects and to support compliance officers in fighting money laundering and financial crime. These are two valuable examples of how the end product of a data science effort can benefit functions along the value chain of a wealth manager.
Overcome your data science challenges
Once your ambitions are translated into use cases, you are ready to start the data science journey. Many wealth managers envisage this as a straightforward, simple undertaking. But just hiring a few data scientists and installing data analytics software might not be enough to harvest all the benefits for your organization.
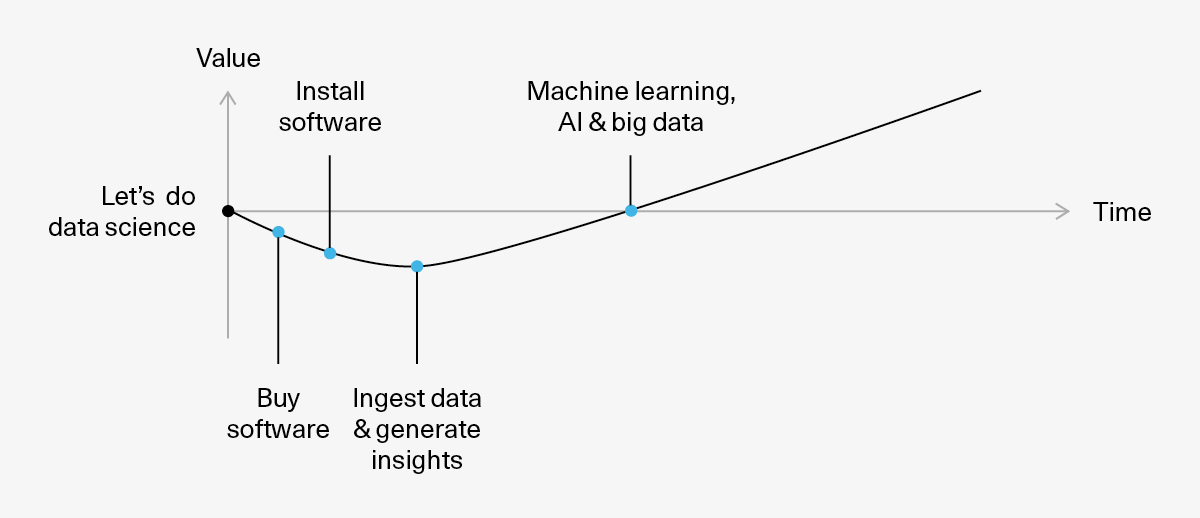
In fact, wealth managers rarely manage to take advantage of the full value of their data, as applying sophisticated analytics methods and algorithms often takes longer than planned. In our view, the following factors frequently challenge and delay the introduction of data science in traditional financial organizations:
Achieving organizational commitment
Make it a top priority of your leadership and the entire organization to transform your business model into a data-driven one. Lone fighters do not achieve results in this discipline. Develop use cases within an agile organizational framework, involving dedicated resources from the front office, back office and compliance.
Mastering technological complexity
The tools and hardware needed to utilize data science are complex and require significant investment. The costs triggered by the installation and maintenance of these complex solutions are often underestimated by financial organizations.
Finding data scientists
Data scientists remain one of the most in-demand job profiles, and many recruiters underestimate the challenge of finding people with the right skill set and experience.
Controlling infrastructure costs
The data centres of many financial institutions have severe space restrictions, leading operators to opt for more sophisticated and expensive space-saving servers. The requirements of big data software are likely to increase demands on the infrastructure and drive costs exorbitantly if not actively managed.
Cleaning up messy data
The effort needed to understand the oddities and nuances of incomplete, messy data in order to clean it up is said to be the biggest pain point in the life of data scientists. Banks are particularly prone to bad data quality, especially when client profiling information has been tracked in free text format over decades. The challenge of making this data ready for use in advanced analytics is often only overcome with the help of machine learning techniques.
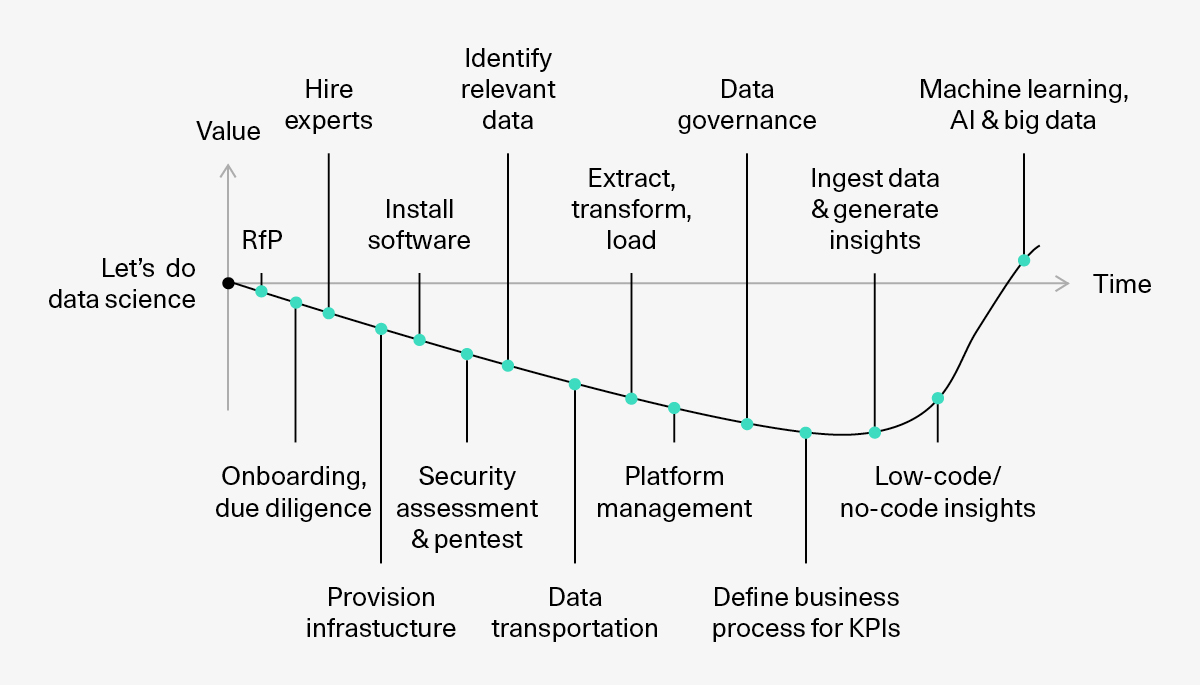
Make data science work for your wealth management
Recognizing and accepting the inherent complexity of data science undertakings is an important foundation to get your use cases off the ground. Luckily, several steps can help a wealth manager overcome this complexity and shorten the resulting project cycles. In our report “Wealth management redefined using artificial intelligence”, we listed three of these steps:
- Build a strategic AI roadmap
- Overcome integration complexity with a powerful service partner
- Profit from the deep learning effect
Read our report to get a set of three workable use cases, in which data is transformed into benefits for a financial organization, and learn how to overcome data science challenges and circumvent common obstacles in wealth management.